What is meant by predictive maintenance?
Predictive maintenance (PdM) is a condition-based maintenance type, utilizing monitoring tools and sensor devices to oversee assets’ status, health, and performance. With PdM, industrial entities can efficiently predict undesired events, securing operational continuity.
Why predictive maintenance is important in manufacturing?
Industries administer valuable, often hard-to-replace assets. Ensuring the longest possible functioning and a fully valid state of machinery, equipment, and production lines are vital for every business operation.
PdM enables the anticipation of events, which translates not only into the prevention of manufacturing line downtimes and extension of equipment life. The approach also allows for efficient planning and optimization of repairs and maintenance.
Assuring proper machine functioning involves monitoring their current status (sensors, etc.), management and monitoring of scheduled components or fluid replacements (examples of time-based maintenance). A combination of PdM, current component condition, with TBM, time since last replacement, and the total number of cycles since last replacement (depending on what the manufacturer recommends, e.g. 1,000 cycles but no more than 2 months or until visible wear) gives the most comprehensive oversight. Combining the manufacturer’s recommendations with the PdM overlap allows for the highest quality of the prediction. As a result, maintenance experts don’t search for malfunctions in the dark, nor follow recommendations blindly.
In short, PdM extends asset life. When industry professionals gain tools to anticipate possible events, adequate counter-measures can be introduced in time to prevent downtimes. The fewer outages, the more efficient the manufacturing process.
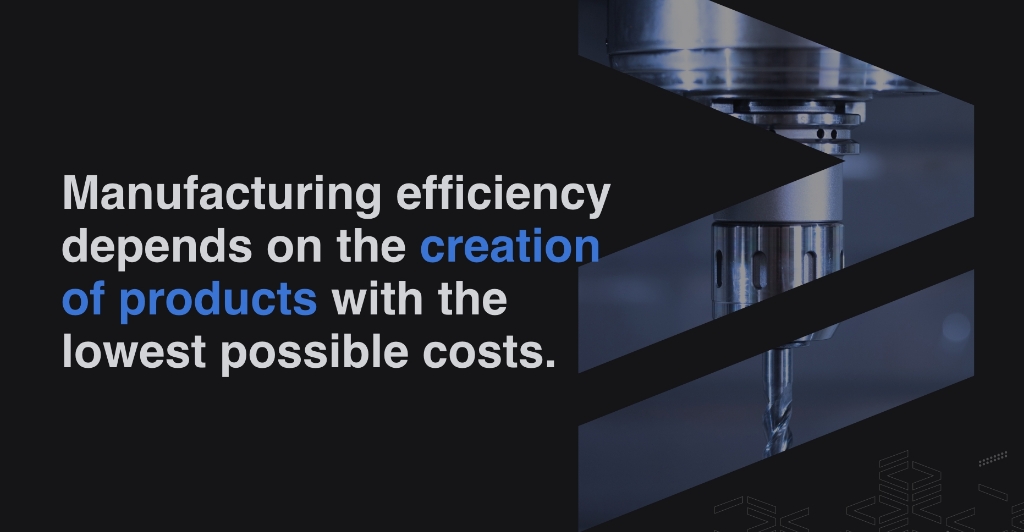
Reducing excessive time, energy, and material waste in the process helps to achieve better results from available resources. And how else can we reduce costs than through being able to predict when that vital production line will malfunction again?
How does predictive maintenance work?
Every working piece of machinery and equipment has its baselines. Implementing predictive maintenance techniques requires observing and analyzing the conditional baselines prior to installing sensors. The starting points act as a foothold for future comparison, once measurements take place. As soon as the monitoring devices are installed and gather data on machines’ operations, noticing abnormalities is simple. Should equipment behave funny, or, to speak in a more industrial manner, perform outside the accepted standards, a predictive maintenance protocol should be triggered.
What is an example of predictive maintenance?
PdM in manufacturing relies on various types of analysis. The most common approaches include:
Acoustic analysis – sonic
A low-cost type of analysis suitable for mechanical machine types working at low speed. Sonic acoustic analysis focuses on proactive lubrication measurement. The technique relies on microphones listening to moving parts and identifying areas requiring attention.
Example: mechanisms moving conveyor belts have their own clashes and trouble areas. Sonic acoustic sensors catch suspicious sounds indicating excessive wear due to lack of gears lubrication.
Acoustic analysis – ultrasonic
Used with both, mechanical and electrical machines. The ultrasonic technique can identify machine friction and stress in ranges inaudible to the human ear. As such, it’s used with more complex equipment to detect subtler sounds than in the case of mechanical machines. Comes at a greater cost than other types of analysis.
Vibration analysis
A cost-effective maintenance technique used mostly for high-rotating machinery. Vibration assessment allows for recognizing looseness and misalignment of elements, bearing wear, and imbalance.
Infrared analysis
Another low-cost type of analysis, suitable for mechanical and electrical machines working at low and high speeds. Most often used for the identification of issues related to motor stress, airflow, and cooling. Allows technicians to recognize problems by assessing electromagnetic radiation with wavelengths longer than visible light.
Example: manufacturing plants install infrared sensors to e.g. monitor trouble areas along the conveyor belt. Observing whether the part of the production line changes temperature outside of acceptable ranges helps prevent overheating and overusing elements.
Oil analysis
A monitoring process requiring analysis of machine oil extracted from the equipment and tested for undesired findings, e.g. presence of water, impurities, or wear particles. Most widely used in the transportation and automotive industries.
Each area of manufacturing has its specific and preferred types of maintenance, depending on the processes and machines used. In the end, they all boil down to one thing – eliminating errors and failures.
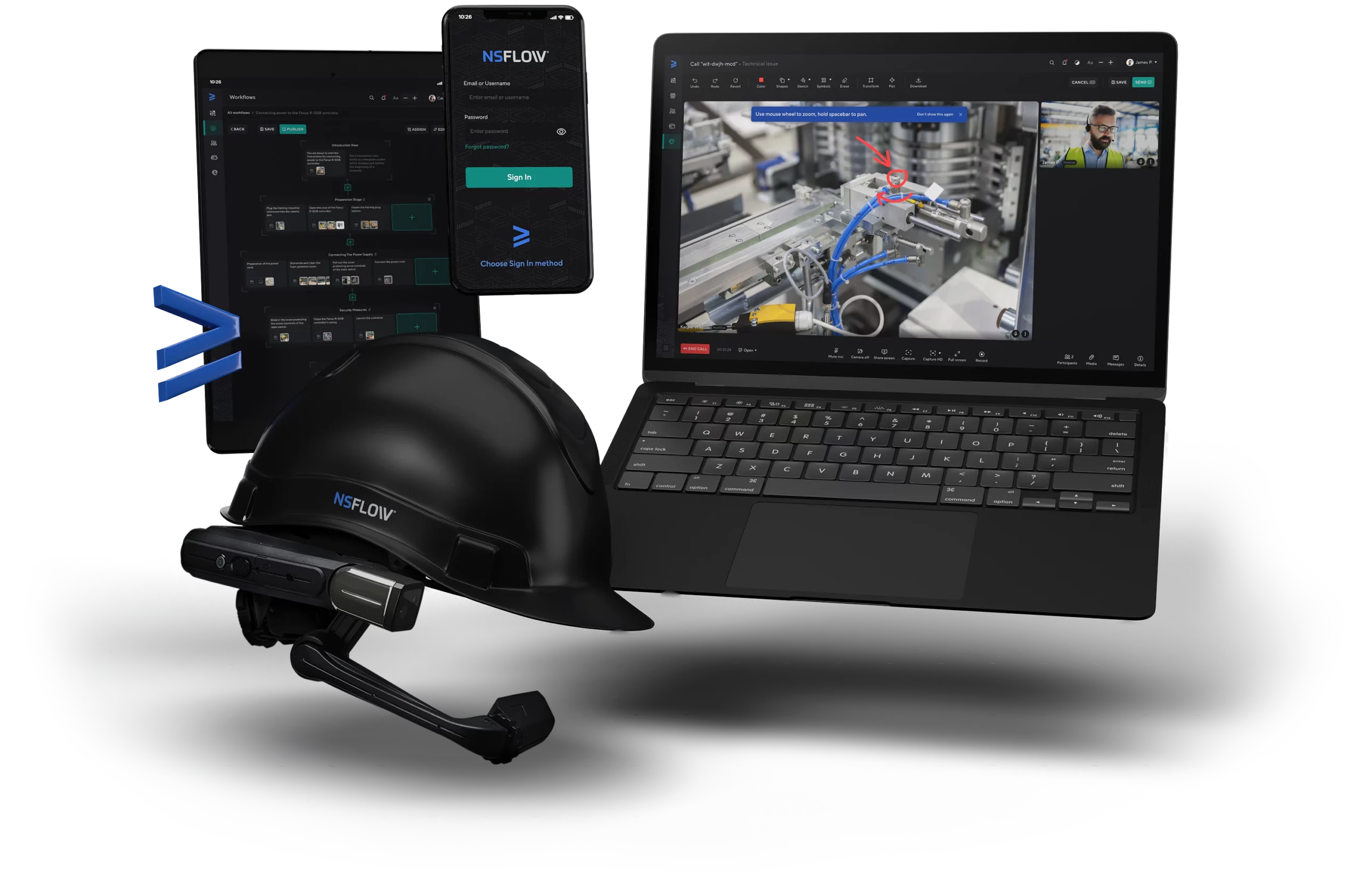
presentation to try
Nsflow in action
Augmented reality in predictive maintenance
AR streamlines the majority of industrial processes, and predictive maintenance is no exception. Frontline workers equipped with wearable devices (or, in some cases, smartphones and tablets) perform asset monitoring on-site. While performing health and status checks, technicians can call up historical data to observe if any divergences occur. Additionally, wearable devices are perfect for capturing the alarming behavior of machinery, allowing personnel to record service and inspection duties.
Augmented reality in industrial maintenance reduces the amount of poorly timed overhauls and applies necessary care when and where it’s most needed to maintain operational continuity. AR is a fast-track to PdM, turning strings of data into accessible and easy-to-view detailed information, available at the touch of a button (or a few voice commands). The location, including those hazardous, is not relevant thanks to RealWear devices.
Real-life predictive maintenance examples:
The line worker scenario
Imagine you’re working with a very expensive and complex machine. You know its operation inside out, but you’re not the technician who repairs it. You can’t “look under the hood” and make sure everything works. One day you arrive at the workstation as you do every day, this time carrying a device in your hand with the new PdM system app installed. It might be a tablet that’s available at the workstation, a company phone, or a RealWear wearable device. Before starting work, you want to make sure everything is running well and the machine won’t cause you any harm, or worse, its failure won’t halt production for days. You launch the app, scan the QR code, enter the machine code, or simply scan the area around you and the system already knows where you are and what you are working with.
You can see when the service was carried out, when the oils were changed, and how much longer until the next check-up. You can make sure that all systems are working to standards. Just as the doctor knows everything about the vital signs of the patient on the operating table, you know everything about the performance of the machine. What if you have a ‘yellow light’? In the event of an abnormality, you don’t need to pull out volumes of manuals and flip through their pages. With AR it’s just a few clicks and a relevant service procedure is set in motion, tailored to your skills with an additional possibility of being connected to an expert or technician. “Red light?” If the repair is considered necessary, it has long since been reported to service technicians and you can carry on with other tasks. No downtimes. “Green light?” You start working on the machine without fear of failure.
What if you still feel something is still wrong? With one click you report the irregularities and the technician-on-duty is on their way.
The technician scenario
The system has notified an inspection/repair so you go to the site. You know exactly what the machine’s operating parameters are, what the reason for the report was, and what needs to be checked or replaced. The system prompts other components that might be an issue, even though no fault has been reported so far. You have a RealWear or Hololens device on, your hands are clad in protective gloves and free to work. With a few commands, the service procedure is launched, guiding you step by step, and reporting in real-time on the state of the machine.
A service note is saved in the system with another single command, and the service/overhaul progress remains in machine history, along with all the details. This way you keep an overview of previous reports, and the machine history will clarify the reason behind submitted issues. What if all systems are working fine, but an employee still reports a problem? You have access to the operator’s comments and insight into the operating parameters as you observe the abnormality. Nothing escapes your notice.
Benefits of predictive maintenance in manufacturing
- Increasing production capacity
- Acquiring precise data
- Extending equipment lifespan
- Reducing equipment replacement costs
- Eliminating unplanned downtimes
- Improving operational efficiency
- Convenience in verifying repair success
- Improving compliance
While predictive maintenance goes along with a few challenges to overcome (e.g. in some cases – high costs of implementation), the pros outweigh the cons. Predictive maintenance is one of the keys to sustainable facility management, allowing to maximize uptime and control operational costs. Asset management strategies include both preventive and corrective operations for comprehensive coverage. However, a stronger focus on proactive measures pays off better than a post-factum response to errors.
Can we predict the future of predictive maintenance?
To an extent, yes, we can.
Judging by the current knowledge, the future of predictive technology looks bright. Encompassing more and more elaborate statistical techniques used for making predictions about unknown events will increase in value.
For now, predictive maintenance is the most advanced type of upkeep. While other types of maintenance services have their supporters, approaches like reactive maintenance (conservation performed when needed, possibly only after a downtime occurs) and time-based maintenance (planned conservation, performed according to a premade schedule, not necessarily when really needed) don’t give the accuracy of PdM.
The condition-based maintenance approach is expected to grow due to the immense need of sustaining industrial machinery in good condition. Since the future of manufacturing is digital, ensuring the care of assets is no exception.